Demo - Bias in Word Embedding¶
In this demo, we are going to work with three complete word embeddings at once in the notebook, which will take a lot of memory (~20GB). Therefore, if your machine doesn’t have plenty of RAM, it is possible to perform the analysis either separately for each word embeddings or only on one.
Imports¶
import matplotlib.pylab as plt
from gensim import downloader
from gensim.models import KeyedVectors
Download and Load Word Embeddings¶
Google’s Word2Vec - Google News dataset (100B tokens, 3M vocab, cased, 300d vectors, 1.65GB download)¶
w2v_path = downloader.load('word2vec-google-news-300', return_path=True)
print(w2v_path)
w2v_model = KeyedVectors.load_word2vec_format(w2v_path, binary=True)
/home/users/shlohod/gensim-data/word2vec-google-news-300/word2vec-google-news-300.gz
Facebook’s fastText¶
fasttext_path = downloader.load('fasttext-wiki-news-subwords-300', return_path=True)
print(fasttext_path)
fasttext_model = KeyedVectors.load_word2vec_format(fasttext_path)
/home/users/shlohod/gensim-data/fasttext-wiki-news-subwords-300/fasttext-wiki-news-subwords-300.gz
Stanford’s Glove - Common Crawl (840B tokens, 2.2M vocab, cased, 300d vectors, 2.03 GB download)¶
import os
import gzip
import shutil
from urllib.request import urlretrieve
from zipfile import ZipFile
from pathlib import Path
from gensim.scripts.glove2word2vec import glove2word2vec
GLOVE_PATH = None
if GLOVE_PATH is None:
print('Downloading...')
glove_zip_path, _ = urlretrieve('http://nlp.stanford.edu/data/glove.840B.300d.zip')
glob_dir_path = Path(glove_zip_path).parent
print('Unzipping...')
with ZipFile(glove_zip_path, 'r') as zip_ref:
zip_ref.extractall(str(glob_dir_path))
print('Converting to Word2Vec format...')
glove2word2vec(glob_dir_path / 'glove.840B.300d.txt',
glob_dir_path / 'glove.840B.300d.w2v.txt')
print('Loading...')
glove_model = KeyedVectors.load_word2vec_format(glob_dir_path / 'glove.840B.300d.w2v.txt')
Downloading...
Unzipping...
Converting to Word2Vec format...
Loading...
Bolukbasi Bias Measure and Debiasing¶
Based on: Bolukbasi Tolga, Kai-Wei Chang, James Y. Zou, Venkatesh Saligrama, and Adam T. Kalai. Man is to computer programmer as woman is to homemaker? debiasing word embeddings. NIPS 2016.
from responsibly.we import GenderBiasWE, most_similar
Create a gender bias word embedding object (GenderBiasWE
)¶
w2v_gender_bias_we = GenderBiasWE(w2v_model, only_lower=False, verbose=True)
Identify direction using pca method...
Principal Component Explained Variance Ratio
--------------------- --------------------------
1 0.605292
2 0.127255
3 0.099281
4 0.0483466
5 0.0406355
6 0.0252729
7 0.0232224
8 0.0123879
9 0.00996098
10 0.00834613
Evaluate the Word Embedding¶
w2v_biased_evaluation = w2v_gender_bias_we.evaluate_word_embedding()
Word pairs¶
w2v_biased_evaluation[0]
pearson_r | pearson_pvalue | spearman_r | spearman_pvalue | ratio_unkonwn_words | |
---|---|---|---|---|---|
MEN | 0.682 | 0.00 | 0.699 | 0.00 | 0.000 |
Mturk | 0.632 | 0.00 | 0.656 | 0.00 | 0.000 |
RG65 | 0.801 | 0.03 | 0.685 | 0.09 | 0.000 |
RW | 0.523 | 0.00 | 0.553 | 0.00 | 33.727 |
SimLex999 | 0.447 | 0.00 | 0.436 | 0.00 | 0.100 |
TR9856 | 0.661 | 0.00 | 0.662 | 0.00 | 85.430 |
WS353 | 0.624 | 0.00 | 0.659 | 0.00 | 0.000 |
Plot the projection of the most extreme professions on the gender direction¶
w2v_gender_bias_we.plot_projection_scores();
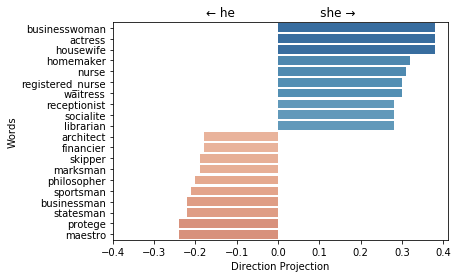
Plot the distribution of projections of the word groups that are being used for the auditing and adjusting the model¶
profession_name - List of profession names, neutral and gender spcific.
neutral_profession_name - List of only neutral profession names.
specific_seed - Seed list of gender specific words.
specific_full - List of the learned specifc gender over all the vocabulary.
specific_full_with_definitional_equalize - specific_full with the words that were used to define the gender direction.
neutral_words - List of all the words in the vocabulary that are not part of specific_full_with_definitional_equalize.
w2v_gender_bias_we.plot_dist_projections_on_direction();
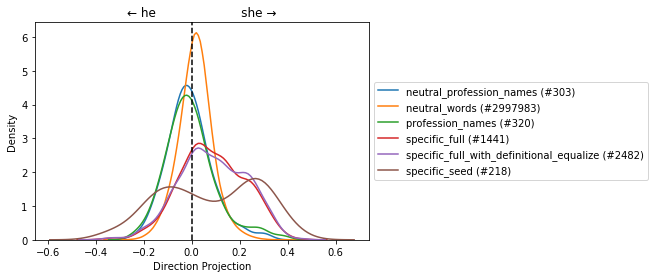
Generate analogies along the gender direction¶
Warning!
The following paper criticize the generating analogies process when
used with unrestricted=False
(as in the original Tolga’s paper):
Nissim, M., van Noord, R., van der Goot, R. (2019). Fair is Better than Sensational: Man is to Doctor as Woman is to Doctor.
Using unrestricted=False
will prevent the generation of analogies
of the form a:x::b:x
such as she:doctor::he:doctor
.
Therefore, the method my introduce “fake” bias.
unrestricted
is set to False
by default.
w2v_gender_bias_we.generate_analogies(131)[100:]
/project/responsibly/responsibly/we/bias.py:528: UserWarning: Not Using unrestricted most_similar may introduce fake biased analogies.
she | he | distance | score | |
---|---|---|---|---|
100 | Michelle | Kris | 0.998236 | 0.278537 |
101 | Marie | Rene | 0.964208 | 0.277007 |
102 | Because | Obviously | 0.831023 | 0.275847 |
103 | Freshman | Rookie | 0.860134 | 0.275070 |
104 | L. | D. | 0.547581 | 0.270656 |
105 | gender | racial | 0.929380 | 0.270301 |
106 | designer | architect | 0.998560 | 0.269882 |
107 | pitcher | starter | 0.880994 | 0.269517 |
108 | dress | garb | 0.922170 | 0.268413 |
109 | midfielder | playmaker | 0.794748 | 0.264263 |
110 | teen | youth | 0.991954 | 0.263156 |
111 | Kim | Lee | 0.912462 | 0.262296 |
112 | wife | nephew | 0.920047 | 0.260974 |
113 | freshmen | rookies | 0.926256 | 0.258481 |
114 | tissue | cartilage | 0.916341 | 0.255413 |
115 | Clinton | Kerry | 0.870915 | 0.253803 |
116 | friend | buddy | 0.778140 | 0.253237 |
117 | Madonna | Jay_Z | 0.992756 | 0.249955 |
118 | sophomore | pounder | 0.994297 | 0.249418 |
119 | French | Frenchman | 0.930501 | 0.245944 |
120 | Hillary_Clinton | Rudy_Giuliani | 0.991420 | 0.245931 |
121 | grandchildren | uncle | 0.977610 | 0.245361 |
122 | designers | architects | 0.958956 | 0.244383 |
123 | amazing | unbelievable | 0.599789 | 0.244071 |
124 | nurses | doctors | 0.860856 | 0.242197 |
125 | incredibly | obviously | 0.995428 | 0.240613 |
126 | designed | engineered | 0.955340 | 0.240351 |
127 | athletes | players | 0.981356 | 0.236221 |
128 | royal | king | 0.975726 | 0.235774 |
129 | cigarette | cigar | 0.874594 | 0.235614 |
130 | Ali | Omar | 0.782018 | 0.235450 |
Let’s examine the analogy #129: she:volleyball::he:football
. We can
try to reproduce it with by applying the arithmetic by ourselves:
football - he + she
with responsibly’s most_similar
:
most_similar(w2v_model, positive=['volleyball', 'he'], negative=['she'], topn=3)
[('volleyball', 0.6795172777227771),
('football', 0.5900704595582971),
('basketball', 0.5792855302799551)]
While gensim’s most_similar
drops results which are in the
original equation (i.e. positives
or negatives
)
w2v_model.most_similar(positive=['volleyball', 'he'], negative=['she'], topn=3)
[('football', 0.5900704860687256),
('basketball', 0.5792855620384216),
('soccer', 0.5567079782485962)]
Because of Tolga’s method of generating analogies which are used in
generate_analogies
, it is not possible to come up with analogies
that have the form she:X::he:X
, that doesn’t reflect a gender bias.
Therefore, the method itself may introduce “fake” bias.
We can run generate_analogies
with unrestricted=True
to address
this issue. Then, for each generated analogies, responsibly’s
most_similar
function will be called twice for she:X::he:Y
-
once for getting X
and second for Y
. An analogy is considered to
be matched if the generated X
and Y
match the results from
most_similar
.
Note: running with unrestricted=True
may take some minutes on the
original word embedding. Therefore we will use a reduced version of
Word2Vec which is available in responsibly.
from responsibly.we import load_w2v_small
model_w2v_small = load_w2v_small()
w2v_small_gender_bias_we = GenderBiasWE(model_w2v_small)
w2v_small_gender_bias_we.generate_analogies(50, unrestricted=True)[40:]
she | he | distance | score | most_x | most_y | match | |
---|---|---|---|---|---|---|---|
40 | sexy | manly | 0.957372 | 0.315748 | manly | sexy | False |
41 | females | males | 0.540050 | 0.309507 | females | males | True |
42 | pink | red | 0.884951 | 0.307515 | red | pink | False |
43 | wonderful | great | 0.685876 | 0.303149 | great | wonderful | False |
44 | chair | chairman | 0.876192 | 0.300693 | chairwoman | chair | False |
45 | friends | buddies | 0.771554 | 0.299829 | buddies | friends | False |
46 | female | male | 0.564742 | 0.295819 | male | female | False |
47 | beauty | grandeur | 0.995714 | 0.293428 | grandeur | beauty | False |
48 | teenager | youngster | 0.726009 | 0.289508 | youngster | teenager | False |
49 | cute | goofy | 0.823867 | 0.289354 | goofy | cute | False |
Generate the Indirect Gender Bias in the direction softball
-football
¶
w2v_gender_bias_we.generate_closest_words_indirect_bias('softball', 'football')
projection | indirect_bias | ||
---|---|---|---|
end | word | ||
softball | bookkeeper | 0.178528 | 0.201158 |
receptionist | 0.158782 | 0.672343 | |
registered_nurse | 0.156625 | 0.287150 | |
waitress | 0.145104 | 0.317842 | |
paralegal | 0.142549 | 0.372737 | |
football | cleric | -0.165978 | 0.017845 |
maestro | -0.180458 | 0.415805 | |
pundit | -0.193208 | 0.101227 | |
businessman | -0.195981 | 0.170079 | |
footballer | -0.337858 | 0.015366 |
Association with percentage of female in occupation¶
import pandas as pd
from responsibly.we.data import OCCUPATION_FEMALE_PRECENTAGE
pd.Series(OCCUPATION_FEMALE_PRECENTAGE).sort_values().plot(kind='barh', figsize=(5, 10));
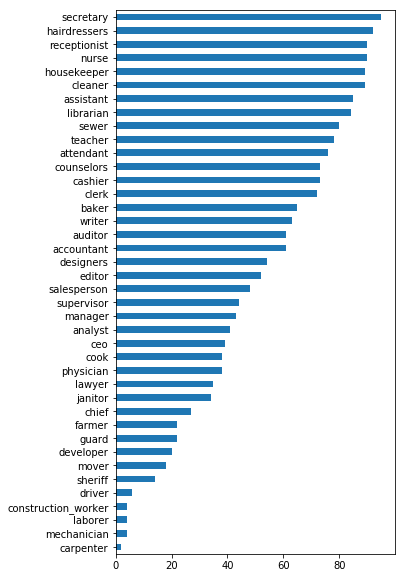
f, ax = plt.subplots(1, figsize=(14, 10))
w2v_gender_bias_we.plot_factual_association(ax=ax);
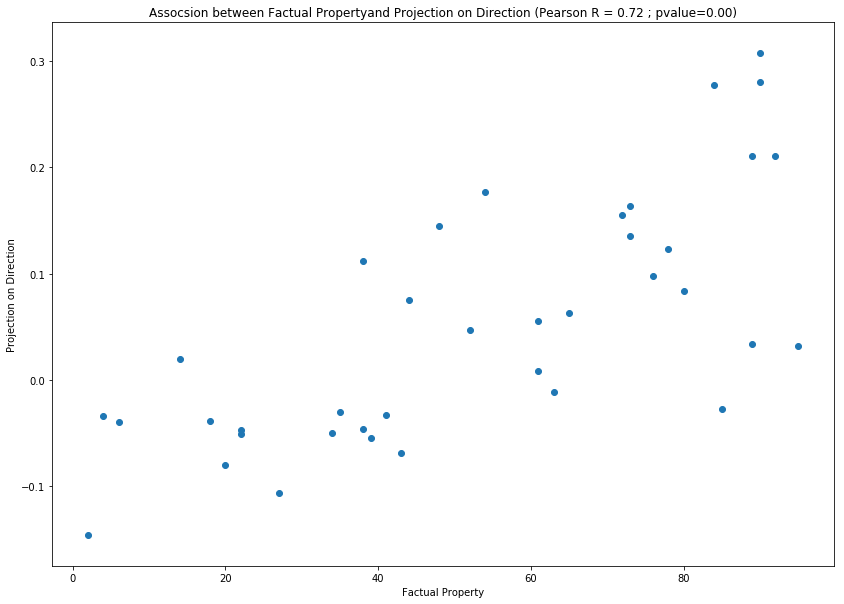
Perform hard-debiasing¶
The table shows the details of the equalize step on the equality sets.
w2v_gender_debias_we = w2v_gender_bias_we.debias('hard', inplace=False)
Neutralize...
100%|████████████████████████████████████████████████████████████████████████| 2997983/2997983 [02:12<00:00, 22549.86it/s]
Equalize...
Equalize Words Data (all equal for 1-dim bias space (direction):
equalized_projected_scalar projected_scalar scaling
------------------------ ---------------------------- ------------------ ---------
(0, 'twin_brother') -0.490669 -0.236034 0.490669
(0, 'twin_sister') 0.490669 0.335621 0.490669
(1, 'she') 0.443113 0.469059 0.443113
(1, 'he') -0.443113 -0.362353 0.443113
(2, 'king') -0.42974 -0.147191 0.42974
(2, 'queen') 0.42974 0.349422 0.42974
(3, 'brother') -0.379581 -0.215975 0.379581
(3, 'sister') 0.379581 0.30764 0.379581
(4, 'SHE') 0.540225 0.385345 0.540225
(4, 'HE') -0.540225 -0.120598 0.540225
(5, 'spokesman') -0.34572 -0.157774 0.34572
(5, 'spokeswoman') 0.34572 0.299861 0.34572
(6, 'son') -0.289697 -0.121614 0.289697
(6, 'daughter') 0.289697 0.292953 0.289697
(7, 'BUSINESSMAN') -0.433807 -0.115595 0.433807
(7, 'BUSINESSWOMAN') 0.433807 0.221015 0.433807
(8, 'prostate_cancer') -0.323124 -0.133314 0.323124
(8, 'ovarian_cancer') 0.323124 0.226155 0.323124
(9, 'SONS') -0.482901 -0.0158731 0.482901
(9, 'DAUGHTERS') 0.482901 0.162073 0.482901
(10, 'SON') -0.521962 0.0122388 0.521962
(10, 'DAUGHTER') 0.521962 0.171916 0.521962
(11, 'boy') -0.29452 -0.0826128 0.29452
(11, 'girl') 0.29452 0.318458 0.29452
(12, 'businessman') -0.433252 -0.218544 0.433252
(12, 'businesswoman') 0.433252 0.379637 0.433252
(13, 'Daughter') 0.469635 0.22278 0.469635
(13, 'Son') -0.469635 -0.168291 0.469635
(14, 'Testosterone') -0.438459 -0.0278472 0.438459
(14, 'Estrogen') 0.438459 0.140597 0.438459
(15, 'Gal') 0.59801 0.110373 0.59801
(15, 'Guy') -0.59801 -0.137855 0.59801
(16, 'He') -0.316178 -0.178255 0.316178
(16, 'She') 0.316178 0.330259 0.316178
(17, 'colt') -0.277647 0.038939 0.277647
(17, 'filly') 0.277647 0.248487 0.277647
(18, 'Uncle') -0.506537 -0.216235 0.506537
(18, 'Aunt') 0.506537 0.347936 0.506537
(19, 'Dad') -0.351847 -0.0842964 0.351847
(19, 'Mom') 0.351847 0.245609 0.351847
(20, 'monastery') -0.41815 0.00771394 0.41815
(20, 'convent') 0.41815 0.264842 0.41815
(21, 'Sons') -0.54096 -0.0837717 0.54096
(21, 'Daughters') 0.54096 0.287506 0.54096
(22, 'her') 0.430368 0.446157 0.430368
(22, 'his') -0.430368 -0.333555 0.430368
(23, 'MALES') -0.357316 0.122686 0.357316
(23, 'FEMALES') 0.357316 0.179751 0.357316
(24, 'Grandfather') -0.404502 -0.106806 0.404502
(24, 'Grandmother') 0.404502 0.266623 0.404502
(25, 'MAN') -0.416802 -0.0911706 0.416802
(25, 'WOMAN') 0.416802 0.257037 0.416802
(26, 'Her') 0.272142 0.267272 0.272142
(26, 'His') -0.272142 -0.122555 0.272142
(27, 'Father') -0.498544 -0.197701 0.498544
(27, 'Mother') 0.498544 0.312446 0.498544
(28, 'SCHOOLBOY') -0.354319 -0.0530171 0.354319
(28, 'SCHOOLGIRL') 0.354319 0.26279 0.354319
(29, 'Males') -0.356464 0.0607782 0.356464
(29, 'Females') 0.356464 0.190471 0.356464
(30, 'Monastery') -0.525956 0.0928539 0.525956
(30, 'Convent') 0.525956 0.210066 0.525956
(31, 'WOMAN') 0.416802 0.416802 0.416802
(31, 'MAN') -0.416802 -0.416802 0.416802
(32, 'gelding') -0.293829 0.0329541 0.293829
(32, 'mare') 0.293829 0.25824 0.293829
(33, 'BROTHER') -0.39397 -0.0290051 0.39397
(33, 'SISTER') 0.39397 0.214365 0.39397
(34, 'NEPHEW') -0.391999 0.0409397 0.391999
(34, 'NIECE') 0.391999 0.133487 0.391999
(35, 'Woman') 0.392149 0.238867 0.392149
(35, 'Man') -0.392149 -0.184176 0.392149
(36, 'Herself') 0.479517 0.29538 0.479517
(36, 'Himself') -0.479517 -0.220471 0.479517
(37, 'FATHERHOOD') -0.391908 -0.00414218 0.391908
(37, 'MOTHERHOOD') 0.391908 0.275103 0.391908
(38, 'HIMSELF') -0.388876 -0.148449 0.388876
(38, 'HERSELF') 0.388876 0.247386 0.388876
(39, 'Dads') -0.413548 0.0235679 0.413548
(39, 'Moms') 0.413548 0.274793 0.413548
(40, 'UNCLE') -0.55611 -0.0985665 0.55611
(40, 'AUNT') 0.55611 0.23314 0.55611
(41, 'EX_GIRLFRIEND') 0.351169 0.0955083 0.351169
(41, 'EX_BOYFRIEND') -0.351169 0.0702349 0.351169
(42, 'KING') -0.492517 0.00505558 0.492517
(42, 'QUEEN') 0.492517 0.189189 0.492517
(43, 'BROTHERS') -0.454497 -0.0106211 0.454497
(43, 'SISTERS') 0.454497 0.231603 0.454497
(44, 'FEMALE') 0.483523 0.179938 0.483523
(44, 'MALE') -0.483523 0.0635581 0.483523
(45, 'CHAIRMAN') -0.41157 -0.0895591 0.41157
(45, 'CHAIRWOMAN') 0.41157 0.115293 0.41157
(46, 'men') -0.37037 -0.0550618 0.37037
(46, 'women') 0.37037 0.344343 0.37037
(47, 'FATHER') -0.391596 -0.0389424 0.391596
(47, 'MOTHER') 0.391596 0.235139 0.391596
(48, 'GIRL') 0.395733 0.242016 0.395733
(48, 'BOY') -0.395733 -0.0573038 0.395733
(49, 'She') 0.316178 0.316178 0.316178
(49, 'He') -0.316178 -0.316178 0.316178
(50, 'grandfather') -0.365996 -0.166732 0.365996
(50, 'grandmother') 0.365996 0.214762 0.365996
(51, 'FELLA') -0.407682 0.0719139 0.407682
(51, 'GRANNY') 0.407682 0.238423 0.407682
(52, 'HER') 0.509812 0.267332 0.509812
(52, 'HIS') -0.509812 -0.0502074 0.509812
(53, 'MOTHER') 0.391596 0.391596 0.391596
(53, 'FATHER') -0.391596 -0.391596 0.391596
(54, 'HIS') -0.509812 -0.509812 0.509812
(54, 'HER') 0.509812 0.509812 0.509812
(55, 'GAL') 0.596187 0.185162 0.596187
(55, 'GUY') -0.596187 -0.0558901 0.596187
(56, 'Brothers') -0.525812 -0.186208 0.525812
(56, 'Sisters') 0.525812 0.283176 0.525812
(57, 'dads') -0.396782 0.0161552 0.396782
(57, 'moms') 0.396782 0.313671 0.396782
(58, 'Congressman') -0.440227 -0.094876 0.440227
(58, 'Congresswoman') 0.440227 0.330899 0.440227
(59, 'Boys') -0.372932 -0.0837174 0.372932
(59, 'Girls') 0.372932 0.265106 0.372932
(60, 'man') -0.346934 -0.220952 0.346934
(60, 'woman') 0.346934 0.340348 0.346934
(61, 'boys') -0.286694 -0.0196151 0.286694
(61, 'girls') 0.286694 0.306264 0.286694
(62, 'kings') -0.451522 -0.150457 0.451522
(62, 'queens') 0.451522 0.284191 0.451522
(63, 'Boy') -0.398915 -0.0954833 0.398915
(63, 'Girl') 0.398915 0.251796 0.398915
(64, 'dudes') -0.420662 -0.0660332 0.420662
(64, 'gals') 0.420662 0.311196 0.420662
(65, 'fatherhood') -0.45719 -0.114388 0.45719
(65, 'motherhood') 0.45719 0.400818 0.45719
(66, 'grandpa') -0.342244 -0.08867 0.342244
(66, 'grandma') 0.342244 0.23426 0.342244
(67, 'girl') 0.29452 0.29452 0.29452
(67, 'boy') -0.29452 -0.29452 0.29452
(68, 'Grandsons') -0.518755 -0.00799486 0.518755
(68, 'Granddaughters') 0.518755 0.280871 0.518755
(69, 'fella') -0.500677 -0.139339 0.500677
(69, 'granny') 0.500677 0.223876 0.500677
(70, 'FATHERS') -0.496495 0.115495 0.496495
(70, 'MOTHERS') 0.496495 0.249673 0.496495
(71, 'FRATERNITY') -0.374105 0.0226484 0.374105
(71, 'SORORITY') 0.374105 0.164613 0.374105
(72, 'MALE') -0.483523 -0.483523 0.483523
(72, 'FEMALE') 0.483523 0.483523 0.483523
(73, 'His') -0.272142 -0.272142 0.272142
(73, 'Her') 0.272142 0.272142 0.272142
(74, 'Chairman') -0.47138 -0.171282 0.47138
(74, 'Chairwoman') 0.47138 0.378271 0.47138
(75, 'BOYS') -0.322782 -0.0660134 0.322782
(75, 'GIRLS') 0.322782 0.231138 0.322782
(76, 'he') -0.443113 -0.443113 0.443113
(76, 'she') 0.443113 0.443113 0.443113
(77, 'SPOKESMAN') -0.380453 -0.0499313 0.380453
(77, 'SPOKESWOMAN') 0.380453 0.0706001 0.380453
(78, 'Mother') 0.498544 0.498544 0.498544
(78, 'Father') -0.498544 -0.498544 0.498544
(79, 'BOY') -0.395733 -0.395733 0.395733
(79, 'GIRL') 0.395733 0.395733 0.395733
(80, 'congressman') -0.427999 -0.0735924 0.427999
(80, 'congresswoman') 0.427999 0.260272 0.427999
(81, 'Councilman') -0.397548 -0.127344 0.397548
(81, 'Councilwoman') 0.397548 0.343178 0.397548
(82, 'GRANDSON') -0.329135 -0.0209265 0.329135
(82, 'GRANDDAUGHTER') 0.329135 0.13067 0.329135
(83, 'male') -0.336739 0.083992 0.336739
(83, 'female') 0.336739 0.282941 0.336739
(84, 'King') -0.496294 -0.116789 0.496294
(84, 'Queen') 0.496294 0.245944 0.496294
(85, 'nephew') -0.34779 -0.199274 0.34779
(85, 'niece') 0.34779 0.251294 0.34779
(86, 'Fatherhood') -0.523357 -0.0710201 0.523357
(86, 'Motherhood') 0.523357 0.348078 0.523357
(87, 'prince') -0.417368 -0.009603 0.417368
(87, 'princess') 0.417368 0.316339 0.417368
(88, 'PRINCE') -0.450062 -0.00160602 0.450062
(88, 'PRINCESS') 0.450062 0.293144 0.450062
(89, 'Colt') -0.611685 -0.148979 0.611685
(89, 'Filly') 0.611685 0.228734 0.611685
(90, 'WIVES') -0.415113 0.0981047 0.415113
(90, 'HUSBANDS') 0.415113 0.19312 0.415113
(91, 'dad') -0.365253 -0.115006 0.365253
(91, 'mom') 0.365253 0.281311 0.365253
(92, 'gal') 0.51301 0.400741 0.51301
(92, 'guy') -0.51301 -0.326011 0.51301
(93, 'father') -0.332768 -0.147961 0.332768
(93, 'mother') 0.332768 0.300389 0.332768
(94, 'Schoolboy') -0.451227 -0.131485 0.451227
(94, 'Schoolgirl') 0.451227 0.241867 0.451227
(95, 'MARY') 0.356334 0.222307 0.356334
(95, 'JOHN') -0.356334 -0.0818515 0.356334
(96, 'wives') -0.402256 0.0785034 0.402256
(96, 'husbands') 0.402256 0.264852 0.402256
(97, 'Gelding') -0.543137 0.0701695 0.543137
(97, 'Mare') 0.543137 0.147159 0.543137
(98, 'Grandpa') -0.389706 -0.088718 0.389706
(98, 'Grandma') 0.389706 0.293524 0.389706
(99, 'chairman') -0.436074 -0.182156 0.436074
(99, 'chairwoman') 0.436074 0.388825 0.436074
(100, 'himself') -0.401098 -0.38296 0.401098
(100, 'herself') 0.401098 0.378141 0.401098
(101, 'GENTLEMEN') -0.504079 -0.0460637 0.504079
(101, 'LADIES') 0.504079 0.191514 0.504079
(102, 'DAUGHTER') 0.521962 0.521962 0.521962
(102, 'SON') -0.521962 -0.521962 0.521962
(103, 'Men') -0.429011 -0.0406838 0.429011
(103, 'Women') 0.429011 0.350025 0.429011
(104, 'Grandson') -0.436104 -0.184543 0.436104
(104, 'Granddaughter') 0.436104 0.262283 0.436104
(105, 'CONGRESSMAN') -0.362044 -0.038846 0.362044
(105, 'CONGRESSWOMAN') 0.362044 0.134128 0.362044
(106, 'Dudes') -0.511909 0.108169 0.511909
(106, 'Gals') 0.511909 0.224083 0.511909
(107, 'sons') -0.295192 -0.0671465 0.295192
(107, 'daughters') 0.295192 0.232942 0.295192
(108, 'HERSELF') 0.388876 0.388876 0.388876
(108, 'HIMSELF') -0.388876 -0.388876 0.388876
(109, 'ex_girlfriend') -0.320849 0.0607288 0.320849
(109, 'ex_boyfriend') 0.320849 0.184493 0.320849
(110, 'herself') 0.401098 0.401098 0.401098
(110, 'himself') -0.401098 -0.401098 0.401098
(111, 'males') -0.311424 0.0583918 0.311424
(111, 'females') 0.311424 0.251908 0.311424
(112, 'mother') 0.332768 0.332768 0.332768
(112, 'father') -0.332768 -0.332768 0.332768
(113, 'HE') -0.540225 -0.540225 0.540225
(113, 'SHE') 0.540225 0.540225 0.540225
(114, 'uncle') -0.343917 -0.184815 0.343917
(114, 'aunt') 0.343917 0.227356 0.343917
(115, 'Fella') -0.635087 -0.0236144 0.635087
(115, 'Granny') 0.635087 0.229781 0.635087
(116, 'councilman') -0.357917 -0.0988956 0.357917
(116, 'councilwoman') 0.357917 0.327105 0.357917
(117, 'schoolboy') -0.401826 -0.185449 0.401826
(117, 'schoolgirl') 0.401826 0.285677 0.401826
(118, 'fraternity') -0.439336 -0.126512 0.439336
(118, 'sorority') 0.439336 0.312585 0.439336
(119, 'MONASTERY') -0.476844 0.0618239 0.476844
(119, 'CONVENT') 0.476844 0.0779661 0.476844
(120, 'KINGS') -0.506902 -0.0580522 0.506902
(120, 'QUEENS') 0.506902 0.0699294 0.506902
(121, 'GRANDPA') -0.38419 0.0151106 0.38419
(121, 'GRANDMA') 0.38419 0.205817 0.38419
(122, 'Gentlemen') -0.556283 -0.0475263 0.556283
(122, 'Ladies') 0.556283 0.247173 0.556283
(123, 'catholic_priest') -0.491534 -0.0380949 0.491534
(123, 'nun') 0.491534 0.26478 0.491534
(124, 'Brother') -0.521082 -0.241616 0.521082
(124, 'Sister') 0.521082 0.345172 0.521082
(125, 'mary') 0.444525 0.192964 0.444525
(125, 'john') -0.444525 -0.0204266 0.444525
(126, 'DADS') -0.583846 0.0983991 0.583846
(126, 'MOMS') 0.583846 0.234249 0.583846
(127, 'Wives') -0.540185 0.104208 0.540185
(127, 'Husbands') 0.540185 0.153448 0.540185
(128, 'Male') -0.405189 0.0366158 0.405189
(128, 'Female') 0.405189 0.198377 0.405189
(129, 'Prince') -0.511457 -0.0959666 0.511457
(129, 'Princess') 0.511457 0.337395 0.511457
(130, 'Mary') 0.488704 0.301974 0.488704
(130, 'John') -0.488704 -0.251126 0.488704
(131, 'Female') 0.405189 0.405189 0.405189
(131, 'Male') -0.405189 -0.405189 0.405189
(132, 'DAD') -0.548123 -0.00731271 0.548123
(132, 'MOM') 0.548123 0.143416 0.548123
(133, 'Prostate_Cancer') -0.388372 -0.101902 0.388372
(133, 'Ovarian_Cancer') 0.388372 0.203867 0.388372
(134, 'Himself') -0.479517 -0.479517 0.479517
(134, 'Herself') 0.479517 0.479517 0.479517
(135, 'female') 0.336739 0.336739 0.336739
(135, 'male') -0.336739 -0.336739 0.336739
(136, 'Girl') 0.398915 0.398915 0.398915
(136, 'Boy') -0.398915 -0.398915 0.398915
(137, 'Man') -0.392149 -0.392149 0.392149
(137, 'Woman') 0.392149 0.392149 0.392149
(138, 'Son') -0.469635 -0.469635 0.469635
(138, 'Daughter') 0.469635 0.469635 0.469635
(139, 'Gentleman') -0.64165 -0.107577 0.64165
(139, 'Lady') 0.64165 0.331577 0.64165
(140, 'woman') 0.346934 0.346934 0.346934
(140, 'man') -0.346934 -0.346934 0.346934
(141, 'his') -0.430368 -0.430368 0.430368
(141, 'her') 0.430368 0.430368 0.430368
(142, 'grandsons') -0.324771 -0.0453894 0.324771
(142, 'granddaughters') 0.324771 0.23981 0.324771
(143, 'daughter') 0.289697 0.289697 0.289697
(143, 'son') -0.289697 -0.289697 0.289697
(144, 'Nephew') -0.589035 -0.104864 0.589035
(144, 'Niece') 0.589035 0.129172 0.589035
(145, 'COLT') -0.574654 0.0448574 0.574654
(145, 'FILLY') 0.574654 0.128587 0.574654
(146, 'fathers') -0.418326 -0.0428798 0.418326
(146, 'mothers') 0.418326 0.363402 0.418326
(147, 'Fraternity') -0.444296 -0.0604545 0.444296
(147, 'Sorority') 0.444296 0.255009 0.444296
(148, 'Fathers') -0.497203 -0.0100986 0.497203
(148, 'Mothers') 0.497203 0.278392 0.497203
(149, 'PROSTATE_CANCER') -0.366725 0.0937956 0.366725
(149, 'OVARIAN_CANCER') 0.366725 0.105068 0.366725
(150, 'GENTLEMAN') -0.415516 0.0409928 0.415516
(150, 'LADY') 0.415516 0.232875 0.415516
(151, 'Businessman') -0.47819 -0.219352 0.47819
(151, 'Businesswoman') 0.47819 0.316095 0.47819
(152, 'GRANDFATHER') -0.331654 0.000438667 0.331654
(152, 'GRANDMOTHER') 0.331654 0.197366 0.331654
(153, 'gentlemen') -0.471394 -0.0597054 0.471394
(153, 'ladies') 0.471394 0.31663 0.471394
(154, 'brothers') -0.401082 -0.199109 0.401082
(154, 'sisters') 0.401082 0.332171 0.401082
(155, 'MEN') -0.504133 0.0498845 0.504133
(155, 'WOMEN') 0.504133 0.268836 0.504133
(156, 'grandson') -0.29271 -0.0966837 0.29271
(156, 'granddaughter') 0.29271 0.240935 0.29271
(157, 'DUDES') -0.547787 0.0688136 0.547787
(157, 'GALS') 0.547787 0.182588 0.547787
(158, 'Kings') -0.569429 -0.058123 0.569429
(158, 'Queens') 0.569429 0.00867156 0.569429
(159, 'testosterone') -0.411566 -0.0294625 0.411566
(159, 'estrogen') 0.411566 0.237813 0.411566
(160, 'Spokesman') -0.402475 -0.128433 0.402475
(160, 'Spokeswoman') 0.402475 0.313343 0.402475
(161, 'Ex_Girlfriend') -0.333678 -0.0357934 0.333678
(161, 'Ex_Boyfriend') 0.333678 0.191153 0.333678
(162, 'gentleman') -0.504986 -0.138084 0.504986
(162, 'lady') 0.504986 0.351432 0.504986
Now our model is gender debiased, let’s check what changed…¶
Evaluate the debiased model¶
The evaluation of the word embedding did not change so much because of the debiasing:
w2v_debiased_evaluation = w2v_gender_debias_we.evaluate_word_embedding()
w2v_debiased_evaluation[0]
pearson_r | pearson_pvalue | spearman_r | spearman_pvalue | ratio_unkonwn_words | |
---|---|---|---|---|---|
MEN | 0.680 | 0.000 | 0.698 | 0.00 | 0.000 |
Mturk | 0.633 | 0.000 | 0.657 | 0.00 | 0.000 |
RG65 | 0.800 | 0.031 | 0.685 | 0.09 | 0.000 |
RW | 0.522 | 0.000 | 0.552 | 0.00 | 33.727 |
SimLex999 | 0.450 | 0.000 | 0.439 | 0.00 | 0.100 |
TR9856 | 0.660 | 0.000 | 0.661 | 0.00 | 85.430 |
WS353 | 0.621 | 0.000 | 0.657 | 0.00 | 0.000 |
w2v_debiased_evaluation[1]
score | |
---|---|
0.737 | |
MSR-syntax | 0.737 |
Calculate direct gender bias¶
w2v_gender_debias_we.calc_direct_bias()
1.2674784842026455e-09
The word embedding is not biased any more (in the professions sense).
Plot the projection of the most extreme professions on the gender direction¶
Note that (almost) all of the non-zero projection words are gender specific.
The word teenager have a projection on the gender direction because it was learned mistakenly as a gender-specific word by the linear SVM, and thus it was not neutralized in the debias processes.
The words provost, serviceman and librarian have zero projection on the gender direction.
w2v_gender_debias_we.plot_projection_scores();
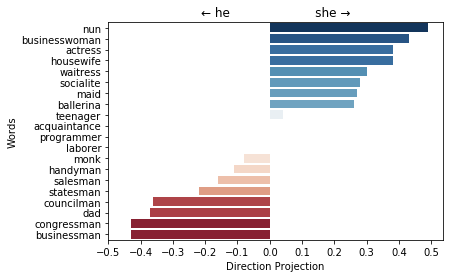
Generate analogies along the gender direction¶
w2v_gender_debias_we.generate_analogies(150)[50:]
/project/responsibly/responsibly/we/bias.py:528: UserWarning: Not Using unrestricted most_similar may introduce fake biased analogies.
she | he | distance | score | |
---|---|---|---|---|
50 | teenagers | males | 0.993710 | 3.133955e-01 |
51 | horses | colt | 0.888134 | 3.126180e-01 |
52 | cousin | younger_brother | 0.758962 | 3.097599e-01 |
53 | really | guys | 0.904382 | 2.780662e-01 |
54 | Lady | Girl | 0.981106 | 2.474099e-01 |
55 | son | Uncle | 0.948682 | 2.285694e-01 |
56 | mum | daddy | 0.980105 | 2.232467e-01 |
57 | Boy | Guy | 0.917343 | 2.170350e-01 |
58 | lady | waitress | 0.972788 | 2.142569e-01 |
59 | boy | gentleman | 0.988562 | 2.129009e-01 |
60 | Hey | dude | 0.919240 | 2.007594e-01 |
61 | striker | lad | 0.981813 | 1.960293e-01 |
62 | father | Father | 0.915928 | 1.809929e-01 |
63 | Cavaliers | Bulls | 0.843798 | 1.757572e-01 |
64 | counterparts | brethren | 0.907441 | 1.688463e-01 |
65 | girlfriend | cousin | 0.902712 | 1.659258e-01 |
66 | God | Him | 0.768831 | 1.635439e-01 |
67 | dealer | salesman | 0.980144 | 1.602965e-01 |
68 | brother | Brother | 0.937209 | 1.509814e-01 |
69 | replied | sir | 0.924511 | 1.454486e-01 |
70 | brothers | Brothers | 0.863473 | 1.444512e-01 |
71 | entrepreneurs | businessmen | 0.870914 | 1.430111e-01 |
72 | muscle | muscular | 0.879145 | 1.414681e-01 |
73 | sons | wives | 0.834242 | 1.283380e-01 |
74 | cancer | prostate | 0.957669 | 1.266430e-01 |
75 | dad | Son | 0.953167 | 1.095110e-01 |
76 | Carl | Earl | 0.907355 | 1.089646e-01 |
77 | Twins | Minnesota_Twins | 0.569007 | 1.061712e-01 |
78 | males | Male | 0.908794 | 1.031754e-01 |
79 | officials | spokesmen | 0.993293 | 1.011937e-01 |
... | ... | ... | ... | ... |
120 | Susan | David | 0.785386 | 3.504101e-08 |
121 | bought | rented | 0.987472 | 3.352761e-08 |
122 | Kevin | AJ | 0.968675 | 3.352761e-08 |
123 | evaluate | monitor | 0.917602 | 3.352761e-08 |
124 | Harvey | Barker | 0.991995 | 3.352761e-08 |
125 | physicians | GPs | 0.930456 | 3.352761e-08 |
126 | Amanda | Adrian | 0.953031 | 3.352761e-08 |
127 | And | Some | 0.952518 | 3.352761e-08 |
128 | Suns | Padres | 0.993828 | 3.352761e-08 |
129 | Oakland | Bay_Area | 0.880000 | 3.352761e-08 |
130 | soldiers | marines | 0.737734 | 3.352761e-08 |
131 | Osama_bin_Laden | Islamic_extremists | 0.981496 | 3.166497e-08 |
132 | Senate | House_Speaker | 0.987174 | 3.166497e-08 |
133 | Larry | Mel | 0.949718 | 3.166497e-08 |
134 | Of_course | Typically | 0.940184 | 3.166497e-08 |
135 | Pyongyang | uranium_enrichment | 0.973787 | 3.166497e-08 |
136 | earn | garner | 0.942567 | 3.166497e-08 |
137 | Brewers | St._Louis_Cardinals | 0.888862 | 3.166497e-08 |
138 | bin_Laden | Osama | 0.686982 | 3.166497e-08 |
139 | Notre_Dame | Badgers | 0.946028 | 3.166497e-08 |
140 | disease | diseases | 0.660790 | 3.166497e-08 |
141 | horrible | horrendous | 0.563251 | 3.073364e-08 |
142 | flying | fly | 0.802642 | 3.073364e-08 |
143 | April | June | 0.317175 | 3.073364e-08 |
144 | Jeff | Greg | 0.505365 | 3.073364e-08 |
145 | Whether | While | 0.895121 | 2.980232e-08 |
146 | Charlie | Jamie | 0.928001 | 2.980232e-08 |
147 | plunged | slumped | 0.732912 | 2.980232e-08 |
148 | anxious | unhappy | 0.975818 | 2.980232e-08 |
149 | Bulldogs | Gaels | 0.828958 | 2.980232e-08 |
100 rows × 4 columns
Generate the Indirect Gender Bias in the direction softball
-football
¶
w2v_gender_debias_we.generate_closest_words_indirect_bias('softball', 'football')
projection | indirect_bias | ||
---|---|---|---|
end | word | ||
softball | infielder | 0.149894 | 1.517707e-07 |
major_leaguer | 0.113700 | 2.272566e-07 | |
bookkeeper | 0.104209 | 6.543536e-08 | |
patrolman | 0.092638 | 8.575430e-08 | |
investigator | 0.081746 | -1.304292e-08 | |
football | midfielder | -0.153175 | -6.718459e-08 |
lecturer | -0.153629 | 5.327011e-08 | |
vice_chancellor | -0.159645 | -2.232139e-08 | |
cleric | -0.166934 | -1.153845e-08 | |
footballer | -0.325018 | 6.779356e-08 |
Now Let’s Try with fastText¶
fasttext_gender_bias_we = GenderBiasWE(fasttext_model, only_lower=False, verbose=True)
Identify direction using pca method...
Principal Component Explained Variance Ratio
--------------------- --------------------------
1 0.531331
2 0.18376
3 0.089777
4 0.0517856
5 0.0407739
6 0.0328988
7 0.0223339
8 0.0193495
9 0.0143259
10 0.0136648
We can compare the projections of neutral profession names on the gender direction for the two original word embeddings¶
f, ax = plt.subplots(1, figsize=(14, 10))
GenderBiasWE.plot_bias_across_word_embeddings({'Word2Vec': w2v_gender_bias_we,
'FastText': fasttext_gender_bias_we},
ax=ax)
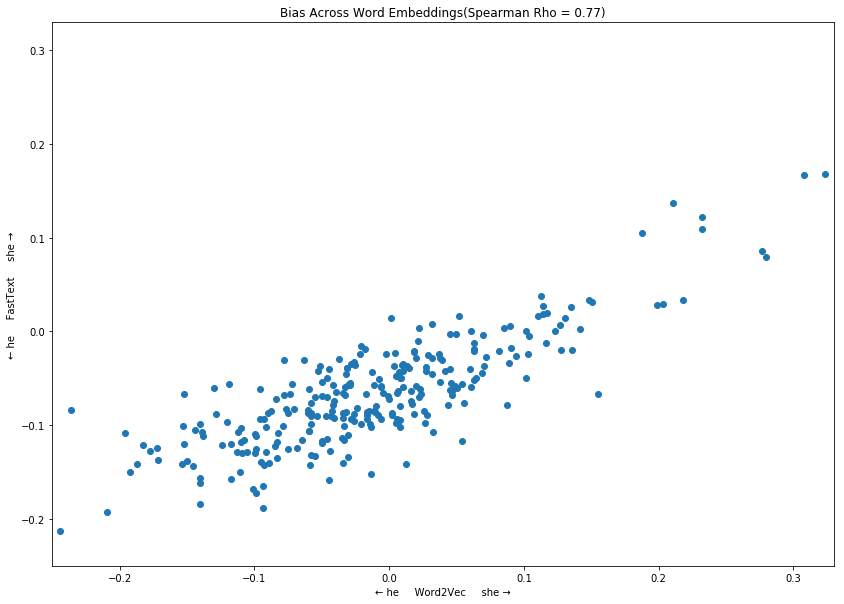
Can we identify race bias? (Exploratory - API may change in a future release)¶
from responsibly.we import BiasWordEmbedding
from responsibly.we.data import BOLUKBASI_DATA
white_common_names = ['Emily', 'Anne', 'Jill', 'Allison', 'Laurie', 'Sarah', 'Meredith', 'Carrie',
'Kristen', 'Todd', 'Neil', 'Geoffrey', 'Brett', 'Brendan', 'Greg', 'Matthew',
'Jay', 'Brad']
black_common_names = ['Aisha', 'Keisha', 'Tamika', 'Lakisha', 'Tanisha', 'Latoya', 'Kenya', 'Latonya',
'Ebony', 'Rasheed', 'Tremayne', 'Kareem', 'Darnell', 'Tyrone', 'Hakim', 'Jamal',
'Leroy', 'Jermaine']
race_bias_we = BiasWordEmbedding(w2v_model,
verbose=True)
race_bias_we._identify_direction('Whites', 'Blacks',
definitional=(white_common_names, black_common_names),
method='sum')
Identify direction using sum method...
neutral_profession_names = race_bias_we._filter_words_by_model(BOLUKBASI_DATA['gender']['neutral_profession_names'])
race_bias_we.calc_direct_bias(neutral_profession_names)
0.0570313461966939
race_bias_we.plot_dist_projections_on_direction({'neutral_profession_names': neutral_profession_names,
'Whites': white_common_names,
'Blacks': black_common_names});
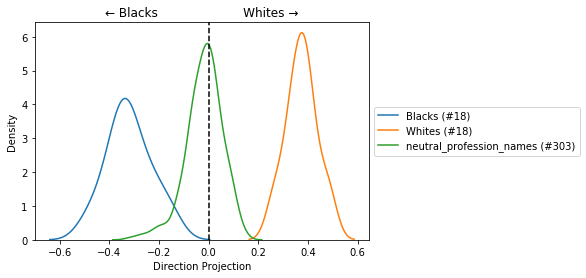
race_bias_we.generate_analogies(30)
/project/responsibly/responsibly/we/bias.py:528: UserWarning: Not Using unrestricted most_similar may introduce fake biased analogies.
Whites | Blacks | distance | score | |
---|---|---|---|---|
0 | white | blacks | 0.984017 | 0.300863 |
1 | central_bank | Federal_Reserve | 0.803605 | 0.288356 |
2 | Everton | Merseyside | 0.977917 | 0.267331 |
3 | Reds | Marlins | 0.913352 | 0.265320 |
4 | Palmer | Lewis | 0.963303 | 0.257839 |
5 | Chapman | Goodman | 0.922701 | 0.257333 |
6 | World_Cup | Olympics | 0.908482 | 0.255429 |
7 | want | Why | 0.981551 | 0.251682 |
8 | virus | HIV | 0.948869 | 0.250508 |
9 | usually | Often | 0.904155 | 0.237194 |
10 | brown | black | 0.920143 | 0.236836 |
11 | definitely | Honestly | 0.990034 | 0.236568 |
12 | soccer | sports | 0.915918 | 0.235413 |
13 | Spurs | Shaq | 0.980871 | 0.235350 |
14 | goalie | shorthanded | 0.997886 | 0.235113 |
15 | tribe | Native_Americans | 0.952653 | 0.234396 |
16 | tournament | regionals | 0.906039 | 0.233398 |
17 | Chile | Latin_America | 0.947953 | 0.232998 |
18 | defendant | Defendant | 0.639279 | 0.230648 |
19 | West_Ham | Londoners | 0.982217 | 0.228847 |
20 | Ghana | Africans | 0.995349 | 0.226636 |
21 | Premier_League | IPL | 0.963885 | 0.226511 |
22 | Leeds | Midlands | 0.924533 | 0.226077 |
23 | Webb | Byrd | 0.976120 | 0.225578 |
24 | shirt | T_shirt | 0.732459 | 0.224453 |
25 | everybody | Somebody | 0.966056 | 0.223887 |
26 | Falcons | Canes | 0.940961 | 0.223731 |
27 | Ferguson | Brown | 0.976292 | 0.222968 |
28 | militants | Militants | 0.758435 | 0.222902 |
29 | Nicholas | Ernest | 0.959587 | 0.221283 |
race_bias_we.generate_analogies(130)[100:]
/project/responsibly/responsibly/we/bias.py:528: UserWarning: Not Using unrestricted most_similar may introduce fake biased analogies.
Whites | Blacks | distance | score | |
---|---|---|---|---|
100 | only | Only | 0.943405 | 0.197555 |
101 | Fletcher | Norris | 0.947846 | 0.197228 |
102 | red | white | 0.928751 | 0.196619 |
103 | jurors | Jurors | 0.697708 | 0.196573 |
104 | complaints | Complaints | 0.710755 | 0.196412 |
105 | knee_injury | ankle_sprain | 0.631131 | 0.196400 |
106 | automatic | automated | 0.955530 | 0.196397 |
107 | worries | Concerns | 0.794042 | 0.196306 |
108 | Player | Athlete | 0.867647 | 0.196244 |
109 | cricket | BCCI | 0.885252 | 0.196228 |
110 | just | Just | 0.951253 | 0.196210 |
111 | Nations | aboriginal | 0.988780 | 0.195973 |
112 | assistant | intern | 0.925927 | 0.195862 |
113 | ##st | nd | 0.899736 | 0.195463 |
114 | Democratic_Party | DNC | 0.994089 | 0.195221 |
115 | game | Game | 0.966801 | 0.195120 |
116 | Bengals | Texans | 0.994674 | 0.194374 |
117 | provincial | Ontario | 0.993721 | 0.194351 |
118 | Scotland | UK | 0.933167 | 0.193891 |
119 | Nathan | Marcus | 0.988859 | 0.193761 |
120 | because | Because | 0.835368 | 0.192906 |
121 | seems | Seems | 0.840152 | 0.192520 |
122 | league | postseason | 0.960766 | 0.192454 |
123 | defender | defenders | 0.715703 | 0.192400 |
124 | celebrations | parades | 0.960279 | 0.192243 |
125 | Republican | Rudy_Giuliani | 0.995810 | 0.192109 |
126 | holiday | Labor_Day | 0.912426 | 0.191855 |
127 | Norway | Oslo | 0.887618 | 0.191662 |
128 | evidence | Evidence | 0.761856 | 0.191556 |
129 | Elementary_School | Public_Schools | 0.940586 | 0.191520 |
f, ax = plt.subplots(figsize=(15, 15))
race_bias_we.plot_projection_scores(neutral_profession_names, 15, ax=ax);
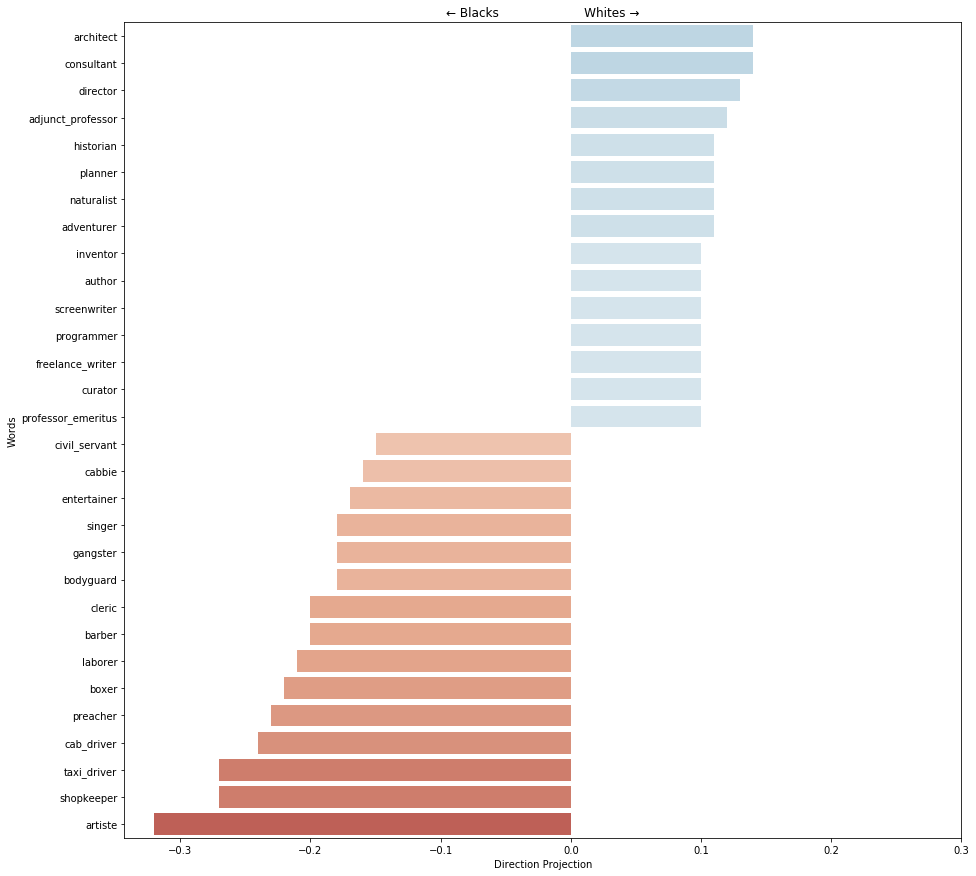
Word Embedding Association Test (WEAT)¶
Based on: Caliskan, A., Bryson, J. J., & Narayanan, A. (2017). Semantics derived automatically from language corpora contain human-like biases. Science, 356(6334), 183-186.
from responsibly.we import calc_all_weat
First, let’s look on a reduced version of Word2Vec.¶
calc_all_weat(model_w2v_small, filter_by='model', with_original_finding=True,
with_pvalue=True, pvalue_kwargs={'method': 'approximate'})
/project/responsibly/responsibly/we/weat.py:368: UserWarning: Given weat_data was filterd by model.
Target words | Attrib. words | Nt | Na | s | d | p | original_N | original_d | original_p | |
---|---|---|---|---|---|---|---|---|---|---|
0 | Flowers vs. Insects | Pleasant vs. Unpleasant | 2x2 | 24x2 | 0.0455987 | 1.2461 | 1.6e-01 | 32 | 1.35 | 1e-8 |
1 | Instruments vs. Weapons | Pleasant vs. Unpleasant | 16x2 | 24x2 | 0.9667 | 1.59092 | 0 | 32 | 1.66 | 1e-10 |
2 | European American names vs. African American n... | Pleasant vs. Unpleasant | 6x2 | 24x2 | 0.136516 | 1.1408 | 2.3e-02 | 26 | 1.17 | 1e-5 |
3 | European American names vs. African American n... | Pleasant vs. Unpleasant | 18x2 | 24x2 | 0.440816 | 1.3369 | 0 | |||
4 | European American names vs. African American n... | Pleasant vs. Unpleasant | 18x2 | 8x2 | 0.33806 | 0.733674 | 1.8e-02 | |||
5 | Male names vs. Female names | Career vs. Family | 1x2 | 8x2 | 0.154198 | 2 | 0 | 39k | 0.72 | < 1e-2 |
6 | Math vs. Arts | Male terms vs. Female terms | 7x2 | 8x2 | 0.161991 | 0.835966 | 5.8e-02 | 28k | 0.82 | < 1e-2 |
7 | Science vs. Arts | Male terms vs. Female terms | 6x2 | 8x2 | 0.303524 | 1.37307 | 7.0e-03 | 91 | 1.47 | 1e-24 |
8 | Mental disease vs. Physical disease | Temporary vs. Permanent | 6x2 | 5x2 | 0.342582 | 1.18702 | 2.5e-02 | 135 | 1.01 | 1e-3 |
9 | Young people’s names vs. Old people’s names | Pleasant vs. Unpleasant | 0x2 | 7x2 | 43k | 1.42 | < 1e-2 |
Let’s reproduce the results from the paper on the full Word2Vec and Glove word embeddings:¶
calc_all_weat(glove_model, filter_by='data', with_original_finding=True,
with_pvalue=True, pvalue_kwargs={'method': 'approximate'})
/project/responsibly/responsibly/we/weat.py:368: UserWarning: Given weat_data was filterd by data.
Target words | Attrib. words | Nt | Na | s | d | p | original_N | original_d | original_p | |
---|---|---|---|---|---|---|---|---|---|---|
0 | Flowers vs. Insects | Pleasant vs. Unpleasant | 25x2 | 25x2 | 2.2382 | 1.5196 | 0 | 32 | 1.35 | 1e-8 |
1 | Instruments vs. Weapons | Pleasant vs. Unpleasant | 25x2 | 25x2 | 2.2906 | 1.5496 | 0 | 32 | 1.66 | 1e-10 |
2 | European American names vs. African American n... | Pleasant vs. Unpleasant | 32x2 | 25x2 | 1.6208 | 1.4163 | 0 | 26 | 1.17 | 1e-5 |
3 | European American names vs. African American n... | Pleasant vs. Unpleasant | 16x2 | 25x2 | 0.7272 | 1.5226 | 0 | |||
4 | European American names vs. African American n... | Pleasant vs. Unpleasant | 16x2 | 8x2 | 0.9177 | 1.3045 | 0 | |||
5 | Male names vs. Female names | Career vs. Family | 8x2 | 8x2 | 1.2670 | 1.8734 | 0 | 39k | 0.72 | < 1e-2 |
6 | Math vs. Arts | Male terms vs. Female terms | 8x2 | 8x2 | 0.1989 | 1.0896 | 1.6e-02 | 28k | 0.82 | < 1e-2 |
7 | Science vs. Arts | Male terms vs. Female terms | 8x2 | 8x2 | 0.3456 | 1.2780 | 2.0e-03 | 91 | 1.47 | 1e-24 |
8 | Mental disease vs. Physical disease | Temporary vs. Permanent | 6x2 | 7x2 | 0.5051 | 1.4442 | 2.0e-03 | 135 | 1.01 | 1e-3 |
9 | Young people’s names vs. Old people’s names | Pleasant vs. Unpleasant | 8x2 | 8x2 | 0.5096 | 1.5520 | 0 | 43k | 1.42 | < 1e-2 |
Results from the paper:¶
glove-weat¶
calc_all_weat(w2v_model, filter_by='model', with_original_finding=True,
with_pvalue=True, pvalue_kwargs={'method': 'approximate'})
/project/responsibly/responsibly/we/weat.py:368: UserWarning: Given weat_data was filterd by model.
Target words | Attrib. words | Nt | Na | s | d | p | original_N | original_d | original_p | |
---|---|---|---|---|---|---|---|---|---|---|
0 | Flowers vs. Insects | Pleasant vs. Unpleasant | 25x2 | 25x2 | 1.4078 | 1.5550 | 0 | 32 | 1.35 | 1e-8 |
1 | Instruments vs. Weapons | Pleasant vs. Unpleasant | 24x2 | 25x2 | 1.7317 | 1.6638 | 0 | 32 | 1.66 | 1e-10 |
2 | European American names vs. African American n... | Pleasant vs. Unpleasant | 47x2 | 25x2 | 0.5672 | 0.6047 | 1.0e-03 | 26 | 1.17 | 1e-5 |
3 | European American names vs. African American n... | Pleasant vs. Unpleasant | 18x2 | 25x2 | 0.4180 | 1.3320 | 0 | |||
4 | European American names vs. African American n... | Pleasant vs. Unpleasant | 18x2 | 8x2 | 0.3381 | 0.7337 | 1.8e-02 | |||
5 | Male names vs. Female names | Career vs. Family | 8x2 | 8x2 | 1.2516 | 1.9518 | 0 | 39k | 0.72 | < 1e-2 |
6 | Math vs. Arts | Male terms vs. Female terms | 8x2 | 8x2 | 0.2255 | 0.9981 | 2.7e-02 | 28k | 0.82 | < 1e-2 |
7 | Science vs. Arts | Male terms vs. Female terms | 8x2 | 8x2 | 0.3572 | 1.2846 | 0 | 91 | 1.47 | 1e-24 |
8 | Mental disease vs. Physical disease | Temporary vs. Permanent | 6x2 | 6x2 | 0.3727 | 1.3259 | 1.3e-02 | 135 | 1.01 | 1e-3 |
9 | Young people’s names vs. Old people’s names | Pleasant vs. Unpleasant | 8x2 | 7x2 | -0.0852 | -0.3721 | 7.4e-01 | 43k | 1.42 | < 1e-2 |
It is possible also to expirements with new target word sets as in this example (Citizen-Immigrant)¶
No WEAT bias in this case.
from responsibly.we import calc_weat_pleasant_unpleasant_attribute
targets = {'first_target': {'name': 'Citizen',
'words': ['citizen', 'citizenship', 'nationality', 'native', 'national', 'countryman',
'inhabitant', 'resident']},
'second_target': {'name': 'Immigrant',
'words': ['immigrant', 'immigration', 'foreigner', 'nonnative', 'noncitizen',
'relocatee', 'newcomer']}}
calc_weat_pleasant_unpleasant_attribute(w2v_model, **targets)
{'Attrib. words': 'Pleasant vs. Unpleasant',
'Na': '25x2',
'Nt': '6x2',
'Target words': 'Citizen vs. Immigrant',
'd': 0.70259565,
'p': 0.13852813852813853,
's': 0.1026485487818718}
Did Tolga’s hard debias method actually remove the gender bias?¶
Warning! The following paper suggests that the current methods have an only superficial effect on the bias in word embeddings:
Gonen, H., & Goldberg, Y. (2019). Lipstick on a Pig: Debiasing Methods Cover up Systematic Gender Biases in Word Embeddings But do not Remove Them. arXiv preprint arXiv:1903.03862.
Note: the numbers are not exactly as in the paper due to a slightly different preprocessing of the word embedding.
First experiment - WEAT before and after debias¶
# before
calc_all_weat(w2v_gender_bias_we.model, weat_data=(5, 6, 7),
filter_by='model', with_original_finding=True,
with_pvalue=True)
/project/responsibly/responsibly/we/weat.py:368: UserWarning: Given weat_data was filterd by model.
Target words | Attrib. words | Nt | Na | s | d | p | original_N | original_d | original_p | |
---|---|---|---|---|---|---|---|---|---|---|
0 | Male names vs. Female names | Career vs. Family | 8x2 | 8x2 | 1.2516 | 1.9518 | 0 | 39k | 0.72 | < 1e-2 |
1 | Math vs. Arts | Male terms vs. Female terms | 8x2 | 8x2 | 0.2255 | 0.9981 | 2.3e-02 | 28k | 0.82 | < 1e-2 |
2 | Science vs. Arts | Male terms vs. Female terms | 8x2 | 8x2 | 0.3572 | 1.2846 | 4.0e-03 | 91 | 1.47 | 1e-24 |
# after
calc_all_weat(w2v_gender_debias_we.model, weat_data=(5, 6, 7),
filter_by='model', with_original_finding=True,
with_pvalue=True)
/project/responsibly/responsibly/we/weat.py:368: UserWarning: Given weat_data was filterd by model.
Target words | Attrib. words | Nt | Na | s | d | p | original_N | original_d | original_p | |
---|---|---|---|---|---|---|---|---|---|---|
0 | Male names vs. Female names | Career vs. Family | 8x2 | 8x2 | 0.7067 | 1.7923 | 0 | 39k | 0.72 | < 1e-2 |
1 | Math vs. Arts | Male terms vs. Female terms | 8x2 | 8x2 | -0.0618 | -1.1726 | 1.0e+00 | 28k | 0.82 | < 1e-2 |
2 | Science vs. Arts | Male terms vs. Female terms | 8x2 | 8x2 | -0.0286 | -0.5306 | 8.4e-01 | 91 | 1.47 | 1e-24 |
For the first experiment, the WEAT score is still significant, while not for the other two. As stated in Gonen et al. paper, this is because the specific gender words as an attribute in the second and the third experiment are handled by the debiasing method.
Let’s use the target words of the first experiment (Male names vs. Female names) with the target words of the other two experiments as attribute words (Math vs. Arts and Science vs. Arts).
from responsibly.we import calc_single_weat
from responsibly.we.data import WEAT_DATA
# Significant result
calc_single_weat(w2v_gender_debias_we.model,
WEAT_DATA[5]['first_target'],
WEAT_DATA[5]['second_target'],
WEAT_DATA[6]['first_target'],
WEAT_DATA[6]['second_target'])
{'Attrib. words': 'Math vs. Arts',
'Na': '8x2',
'Nt': '8x2',
'Target words': 'Male names vs. Female names',
'd': 1.513799,
'p': 0.0009324009324009324,
's': 0.34435559436678886}
# Significant result
calc_single_weat(w2v_gender_debias_we.model,
WEAT_DATA[5]['first_target'],
WEAT_DATA[5]['second_target'],
WEAT_DATA[7]['first_target'],
WEAT_DATA[7]['second_target'])
{'Attrib. words': 'Science vs. Arts',
'Na': '8x2',
'Nt': '8x2',
'Target words': 'Male names vs. Female names',
'd': 1.0226882,
'p': 0.022455322455322457,
's': 0.20674265176057816}
Second experiment - Clustering as classification of the most biased neutral words¶
GenderBiasWE.plot_most_biased_clustering(w2v_gender_bias_we, w2v_gender_debias_we);
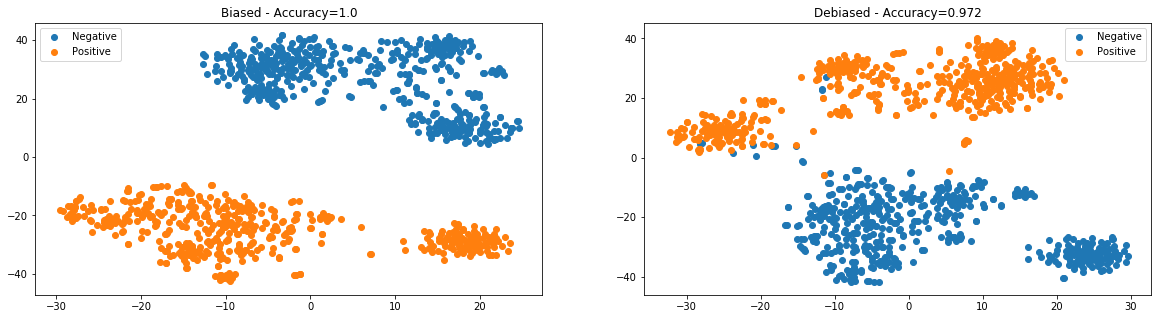